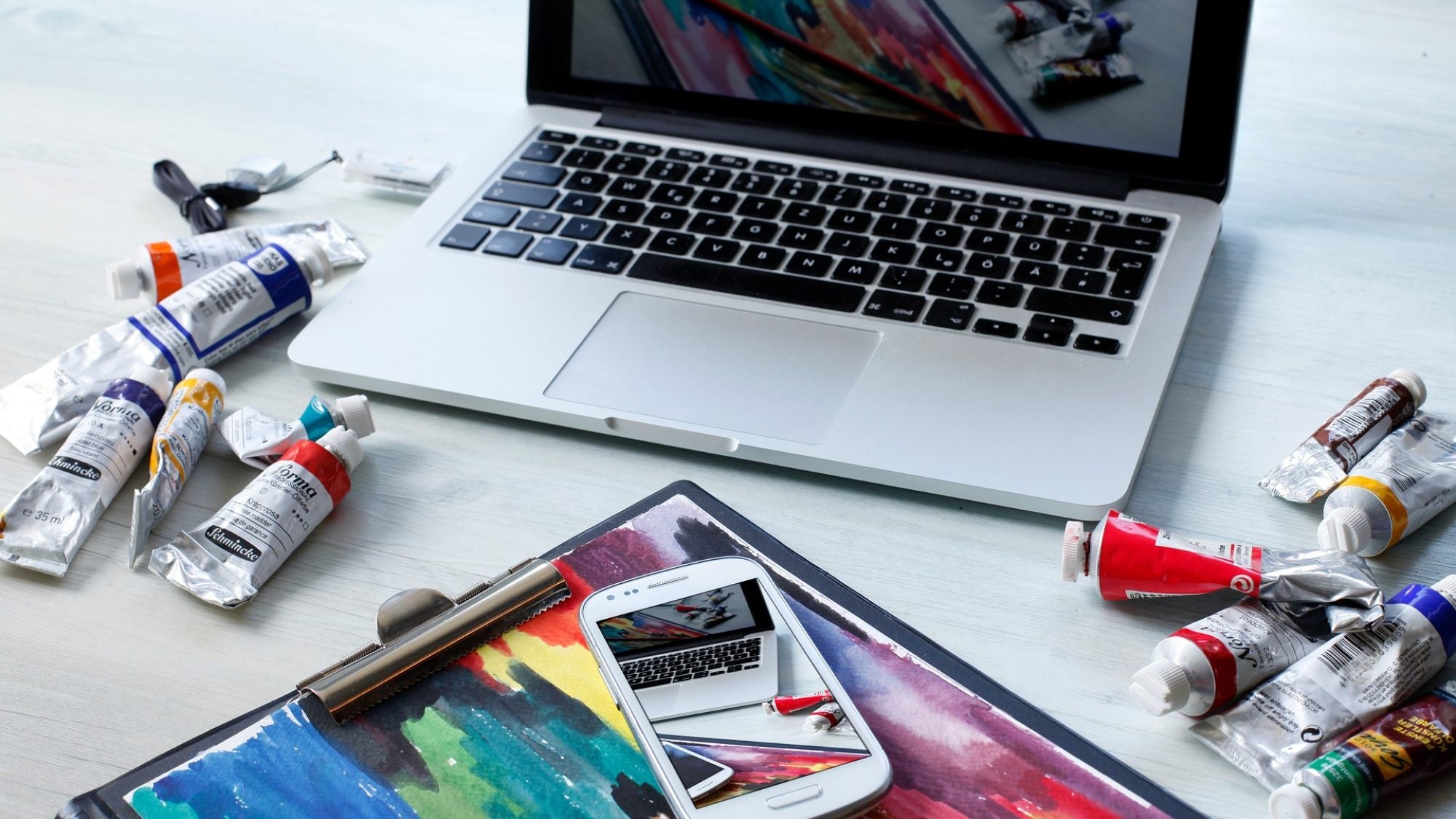
Title: Innovation and Artists’ Rights in the Age of Generative AI
The rise of generative artificial intelligence (AI) in the music industry has sparked a significant debate among artists and AI developers. This technology, which transforms vast datasets into original content, poses significant challenges for copyright law because it often uses copyrighted material without consent, which could lead to potential infringement issues. This article explores three key vectors in this debate: legislation, scarcity, and varied global approaches to AI governance. A balanced, adaptive regulatory framework is essential to support AI innovation while protecting creators’ rights, thus emphasizing the need for global cooperation and fair legal standards.
Introduction
In recent months, artists such as Billie Eilish and Pearl Jam have voiced concerns about generative AI in music, highlighting issues around creativity and artists’ rights. These artists have called on AI developers and digital service providers to halt practices that undermine human creativity and devalue the rights of artists. Specifically, they criticize the training of AI models on unlicensed music, arguing that it infringes upon the rights of artists and floods the market with AI-generated content, severely diluting the earnings for original creators.
Music is one of many creative industries that generative AI has disrupted. AI models, which can be trained on trillions of data points, often incorporate a vast array of copyrighted content—from film scripts and news articles to music—frequently without the copyright owners’ consent. This article examines the transformative impact of generative AI on creative industries, particularly music, where artists have raised concerns about copyright infringement. It argues that balancing innovation and artists’ rights requires reconciling legislation, scarcity of training data, and varied global approaches to AI governance. By exploring current legislative responses, considering the ethical challenges of synthetic data, and comparing regulatory strategies in the United States, European Union (EU), and China, this article emphasizes the need for an adaptive and fair regulatory framework. It concludes with a call for global cooperation and legal reforms to support AI innovation while protecting creators’ rights.
Legislation
By design, generative AI learns from extensive datasets, often encompassing copyrighted materials, to produce new, derivative works. This ability to reproduce distinct, copyrighted content underscores generative AI’s potential for copyright infringement. The fair use doctrine, which allows limited use of copyrighted material without permission for purposes such as criticism, comment, news reporting, teaching, scholarship, or research, is a critical defense used by AI developers. However, the scale and nature of AI’s use of copyrighted materials challenge traditional interpretations of fair use, prompting a need for clearer guidelines that reflect the unique characteristics of AI-generated content and its reliance on pre-existing works.
In response to generative AI’s copyright challenges, the United States is considering the Generative AI Copyright Disclosure Act and state-level measures like Tennessee’s Ensuring Likeness Voice and Image Security (ELVIS) Act to protect artists’ rights and manage AI’s use of copyrighted materials. The first would mandate that AI companies disclose copyrighted works used in the training of their models prior to the model’s release to the public, as well as when any significant updates are made to an existing model’s training dataset. The second offers civil and criminal remedies, including fines and jail time for violations, while also delineating exceptions in accordance with First Amendment rights, ensuring fair use in contexts like news broadcasting, commentary, criticism, and parody.
Scarcity of Training Data
AI’s insatiable data needs have led to a significant shortage of training data—a shortage further exacerbated by the regulatory initiatives described above—pushing companies toward synthetic data solutions despite transparency and ethical concerns. ,. This scarcity has spurred a quest for alternative sources of training data, from publicly available video transcripts to the controversial realm of AI-generated “synthetic data,” or data created using algorithms, statistical models, or generative AI. It mimics real-world data and has the same mathematical properties as the real data it is based on. Companies like Anthropic are exploring the creation of higher-quality synthetic data to circumvent the issues created by regulations and overall shortages.
Relying on AI-generated data could lead to a digital echo chamber, a phenomenon humorously termed “Habsburg AI” by analogy to the genetic pitfalls of inbreeding. This metaphorical inbreeding raises questions about the diversity and reliability of the data that AI models are trained on. In the worst case, Habsburg AI could cause “model collapse,” where AI fails to generalize beyond its narrow, self-referential dataset.
As with the real world, the scarcity of resources also risks exacerbating fault lines and hardening positions on intellectual property issues. AI developers and content creators engaged in acrimonious litigation and legislative lobbying will prioritize their public positions and commitments to constituents at the expense of the common good. This pattern has already stymied patent law reform for over a decade.
Global Approaches
While legal standards are appealing for their promise of neutral, objective guidance in AI governance, the reality is more complex. Like any form of regulation or guideline, laws are shaped by human actors within specific institutional and political contexts. America’s approach is market-driven, where private companies, through their practices and internal frameworks, set de facto standards. The EU’s approach is rights-focused, creating “opt-outs” for copyright owners to remove their works from text and data mining purposes. European legislators have ratified a significant new law demanding clarity on the use of all training data, regardless of its copyright situation. This move, part of the EU’s effort to shape worldwide trends via the “Brussels effect,” introduces a theme of digital autonomy into the regulatory framework. China has taken a state-driven approach and has shown that it favors the copyright protection of AI-created content. It has enacted the earliest and most comprehensive set of rules worldwide for all AI-generated content services. These regulations mandate that developers legally train their models, ensuring they do not violate the intellectual property rights of others.
These three approaches showcase varied global approaches to AI governance—all outgrowths of states’ regulatory “digital empires.” Each approach reflects a direct exercise of state power in shaping market practices and standards, further emphasizing the political dimensions of regulation. The tension between the politics of governance and the quest for objective, universally applicable standards presents a fundamental challenge for AI governance, highlighting the need for global cooperation and international standards. Acknowledging the political nature of governance standards is crucial, as is navigating them through trust-building and a rules-based order.
Synthesis: Adaptability and Fairness
Stakeholders from the entertainment industry and academia at a recent U.S. Patent and Trademark Office and Copyright Office symposium emphasized the inadequacy of current laws in protecting individuals against unauthorized AI-generated content. Participants reached a consensus on the need for federal legislation to provide guidelines for AI content, including on social media platforms. They agreed that laws should require model builders to implement filters or restrictions on AI outputs, and that regulatory bodies should mandate practices that reduce the likelihood of copyright infringement by AI-generated content. By embracing principles of adaptiveness and fairness, legal frameworks can provide robust safeguards for creators’ rights while supporting the continued advancement of generative AI technology.
An adaptive approach should update the law by accounting for AI’s role in content creation, clarifying the application of fair use to AI-generated content, and considering new models of copyright ownership that reflect the collaborative nature of AI-assisted creations. The Generative AI Copyright Disclosure Act and ELVIS Act are examples of such change. However, change must also be rooted in fidelity to existing doctrine.
Matthew Sag offers an example of a doctrinal adaptation that focuses on the lack of expressive substitution. Copyright law, at its core, protects original expression. Activities such as reverse engineering for interoperability, plagiarism detection, and the creation of search engine indexes, despite involving substantial copying, do not infringe upon the essence of copyright as they do not entail expressive substitution. Similarly, while generative AI’s data-hungry models necessitate extensive copying, such copying is not aimed at communicating the original work’s expression to a new audience and should, therefore, fall under fair use.
Beyond these examples, licensing solutions such as direct and collective licensing meet the needs of technology companies, users of copyrighted works, authors, and publishers. Direct licensing offers tailored, flexible agreements suited to specific needs, while collective licensing provides broad, simplified access and efficient administration of rights. Together, they ensure that technology can advance and innovate while respecting and compensating the creators and owners of copyrighted content. For instance, OpenAI has signed content deals with Time magazine, The Atlantic, Vox Media, the Financial Times, Axel Springer, Le Monde, and Prisa Media that would give ChatGPT access to their content.
Managing rights collectively is advantageous when dealing with vast quantities of content from many different owners, especially when training AI systems that use diverse works. At the same time, AI companies are also adapting by exploring ways to make their models “unlearn” copyrighted information and pledging not to use customer-generated data for further training, aiming to minimize the risk of generating infringing content.
Moreover, addressing the fairness of AI systems requires comprehensive regulatory guidelines that ensure AI systems balance innovation with regulation, thereby fostering an environment where AI promotes the public good. A concept of fairness, inspired by Rawlsian principles, can help guide a revised legal framework that addresses economic efficiencies and considers the broader societal impacts of AI-generated content. Rawls’ veil of ignorance encourages individuals to design rules without knowing their own position within that society. In the AI context, policy formulation that starts from the premise of technology and content agnosticism helps ensure rules are fair and impartial, promoting equality and justice for AI developers, creators whose work is used for training, or users.
Legal reform can also incorporate a balancing test that evaluates the benefits and potential harms associated with generative AI. Like the rule of reason in antitrust law, this test can be coupled with less restrictive legal measures that mitigate AI’s adverse effects while leveraging its benefits. Here, Rawls’ difference principle nuances such balancing by arguing that inequalities in society are acceptable only if they benefit the least-advantaged members. In this regard, AI policies should ensure that AI benefits the most disadvantaged groups, including those who may not be capable of vocally vying for their share of the economic pie. Regulations requiring AI-generated profits to contribute to social welfare programs, support underserved communities, and help affected content creators upskill and reskill will help create trust and goodwill for the AI transition without fracturing the social compact.
Finally, the law should also recognize broader concepts of fairness, such as the impact of environmental sustainability. For instance, the relentless pursuit of “bigger and better” models comes at a significant environmental and societal cost, from immense energy consumption to reliance on rare earth minerals. Some observers, like researcher Pablo Villalobos, suggest that the answer lies not in scaling up generative AI outputs but in seeking breakthroughs that allow for more efficient use of data and resources.
Conclusion
Generative AI presents multifaceted challenges and opportunities in creative industries such as music. The scarcity of high-quality training data propels the use of synthetic alternatives, raising ethical and reliability concerns. Additionally, the emergence of diverse global regulatory approaches, from the United States’ market-driven strategies to the EU’s rights-focused policies and China’s state-driven regulations, reflect the complex political and economic landscapes shaping AI governance.
An adaptive and fair regulatory framework that supports generative AI’s growth while ensuring robust protection for creators is necessary to navigate the interplay between creativity, law, and technology, fostering an environment where AI innovation can thrive without compromising the rights of human creators. Such reforms would provide much-needed clarity for creators and developers alike.
. . .
Daryl Lim is the H. Laddie Montague Jr. Chair in Law at Penn State Dickinson Law. He is also the Associate Dean for Research and Innovation and Founding Director of the Intellectual Property Law and Innovation Initiative. At the university level, he is a co-hire at the Institute of Computational and Data Sciences and an affiliate at the Center for Socially Responsible Artificial Intelligence.
Image credit: ptra, Pixabay Content License, via Pixabay.
Recommended Articles
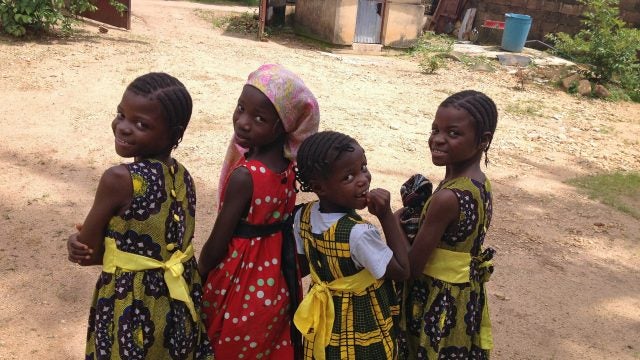
Currently, an estimated 230 million girls and women are living with female genital mutilation (FGM) worldwide, up from 200 million in 2016. While global efforts to eliminate FGM…
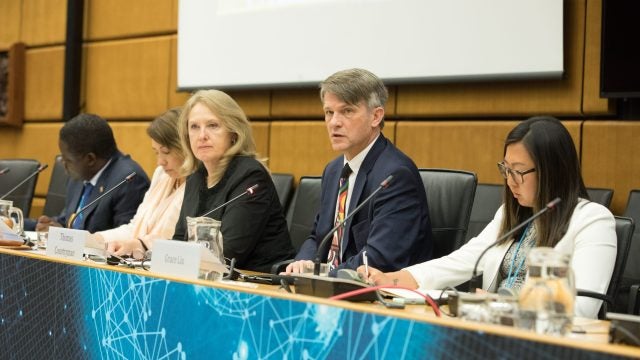
War and conflict have profound effects on society, including the critical fields of science. The paper argues that scientific protectionism, which includes restricting international collaborations and open science, threatens innovation,…
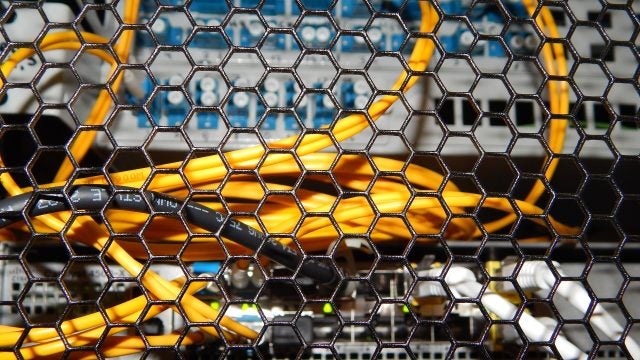
South Korea has faced an onslaught of cyberattacks in recent years, primarily from North Korea, which has employed AI technologies for sophisticated assaults. In response to these attacks, South Korea…